Join us as we explore
The New Frontiers of Machine Learning
Free Virtual Event | March 7-8, 2023
Days of content
Speakers
Attendees online
Join us for this second edition of Convergence Conference on March 7-8, 2023 and explore the new frontiers of machine learning, including novel applications, tools and processes! This two-day virtual event features 25+ talks, panels and workshops by leading data scientists who share cutting-edge ML techniques to solve complex problems in the real world.
About Convergence
Machine learning solutions are increasingly employed by companies across industries to generate economic and social value. The power of algorithms provides firms with valuable insights and enables them to build better products and services at a faster pace and at lower costs. However, the AI journey has just begun and nascent, exciting approaches are being developed to create ML models that are even more reliable, ethical, creative and explainable. Convergence gathers data scientists, ML engineers and researchers to meet each other and discover the latest technology, advancements and trends in ML.
Speakers
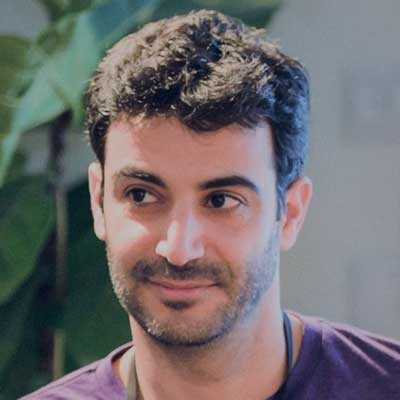
Omri Allouche
Head of Research at Gong
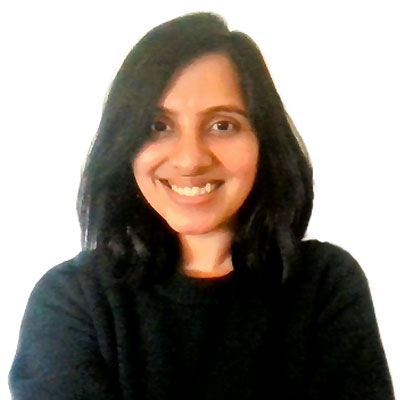
Iswarya Murali
Principal Data Scientist at Microsoft
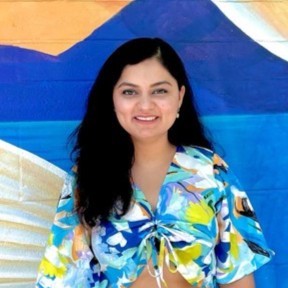
Jigyasa Grover
Senior Machine Learning Engineer at Twitter
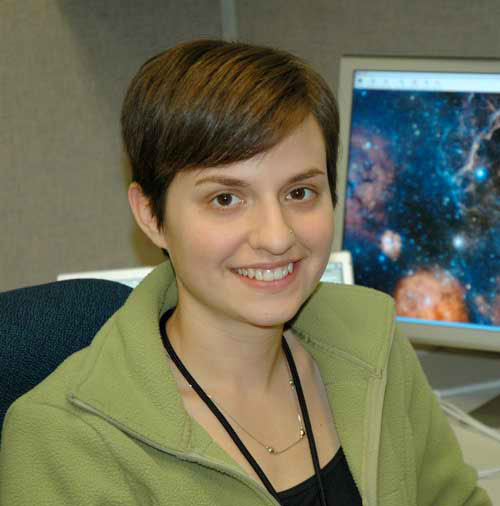
Ali Vanderveld
Senior Staff Scientist at Wayfair
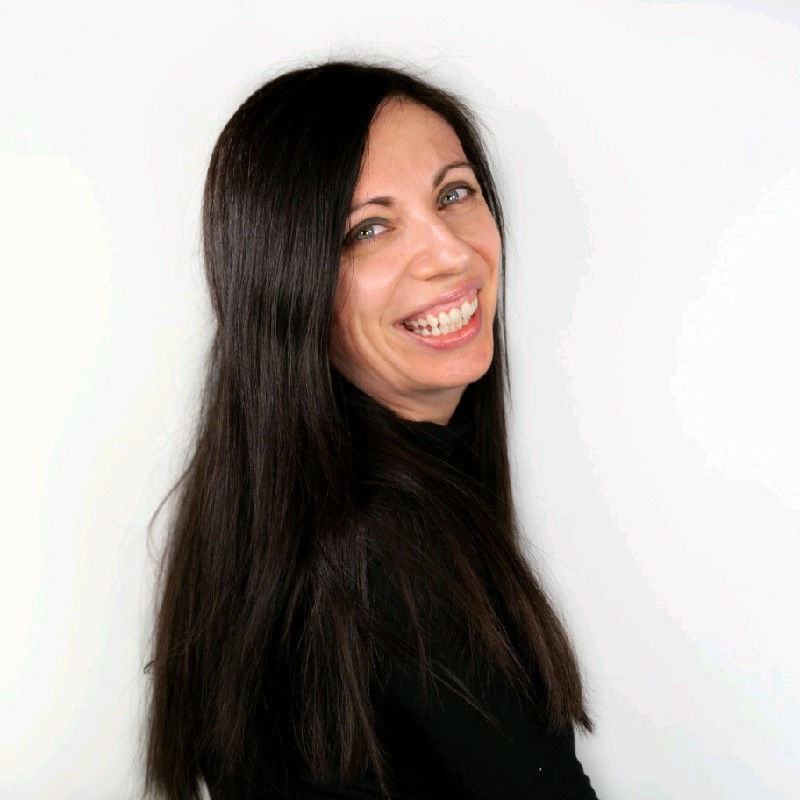
Angelica Lo Duca
Researcher at IIT-CNR
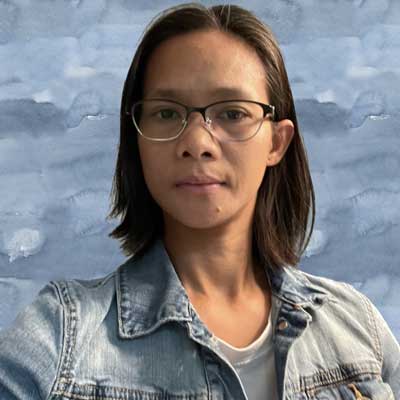
Madonna M Dodd
Principal Data Engineer at Microsoft
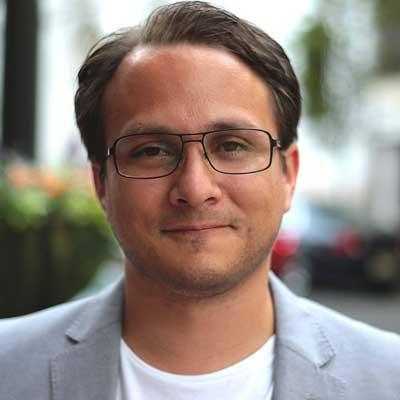
Serg Masís
Climate & Agronomic Data Scientist at Syngenta
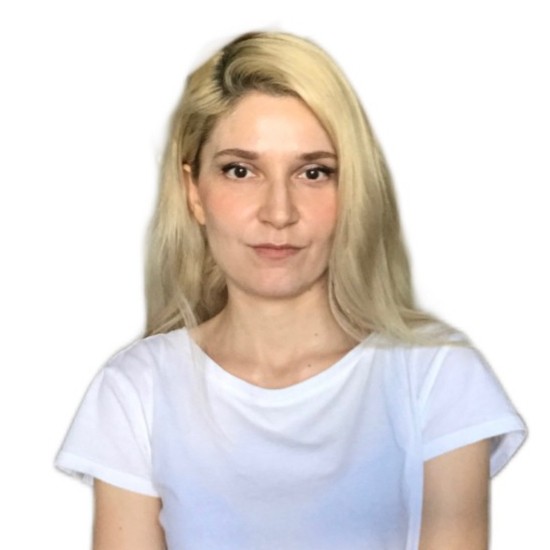
Ozlem Yaylaci
Principal Data Scientist at JLG Industries
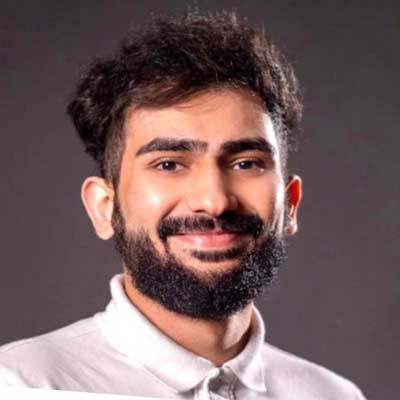
Ameya Diwan
Ethical AI Analyst at Rocket Central
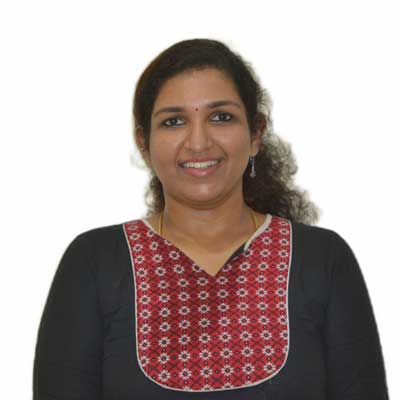
Sandhya Gopalan
AI & MLOps Practice Head at EY Global Delivery Services

Hugo Bowne
Head of Developer Relations at Metaflow/Outerbound
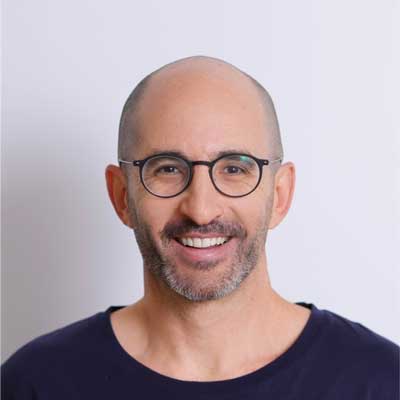
Ronen Dar
CTO and Co-Founder at Run.ai
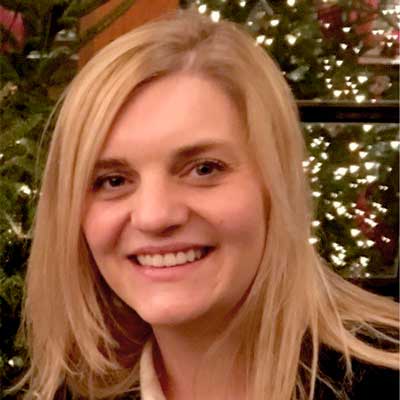
Brittney Monroe
Senior Engineer at Carvana
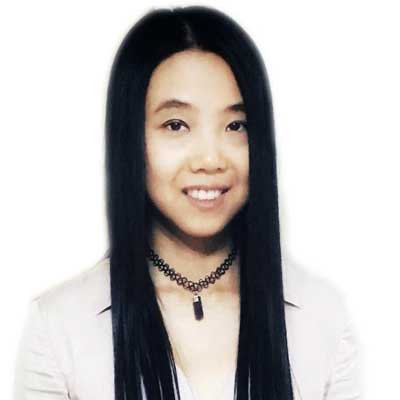
Christina Taylor
Senior Staff Data Engineer at Catalyst Software
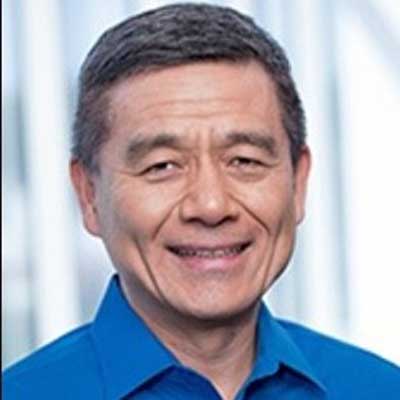
Wei Li
VP & GM, Artificial Intelligence & Analytics at Intel Corporation
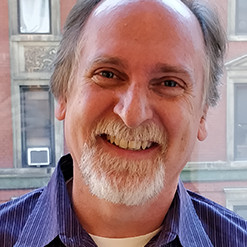
Doug Blank
Head of Research at Comet
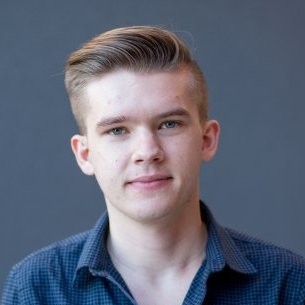
Caleb Kaiser
Software Engineer at Comet
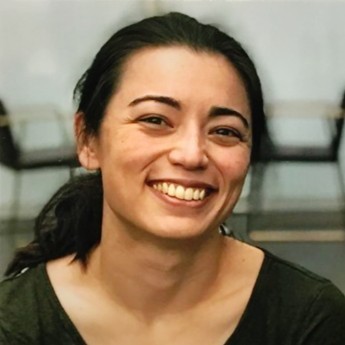
Mikiko Bazeley
Head of MLOps at Featureform

Greig Cowan
Head of Data Science for Data Innovation at NatWest Group

Premalatha Thangamani
Director of Data Science at PayPal
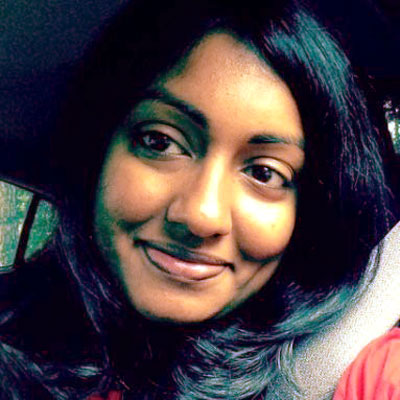
Sharmila Chockalingam
Head of Product Marketing, MLOps at Comet
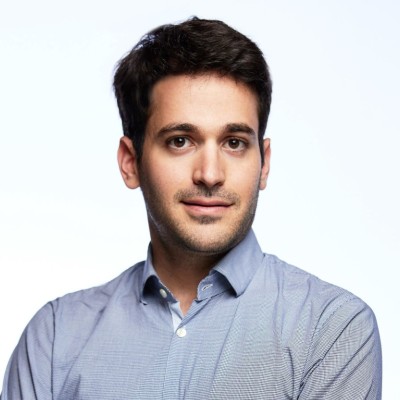
Gideon Mendels
Co-Founder and CEO at Comet
Schedule
Day 1 | Tuesday March 7
12:00pm ET
Introduction
12:05pm ET
The Role of Data Scientists in the Age of GPT and LLMs
Omri Allouche – Head of Research at Gong
In the age of GPT and LLMs, technology companies have the opportunity to revolutionize the way they build products. These advanced models have the potential to greatly speed up the development process, but in order to build products that people actually use and love, a skilled data science team is crucial. In this talk, we will discuss the role of data scientists in this new landscape, explore how they can best make use of GPT and LLMs to drive innovation, and discuss how to best organize data science teams to stay ahead of the curve in the fast-paced world of data science and technology.
12:40pm ET
Sculpting Data for Machine Learning
Jigyasa Grover – Senior Machine Learning Engineer at Twitter
In the contemporary world of machine learning algorithms – “data is the new oil”. For the state-of-the-art ML algorithms to work their magic it’s important to lay a strong foundation with access to relevant data. Volumes of crude data are available on the web nowadays, and all we need are the skills to identify and extract meaningful datasets. This talk aims to present the power of the most fundamental aspect of Machine Learning – Dataset Curation, which often does not get its due limelight. It will also walk the audience through the process of constructing good quality datasets as done in formal settings with a simple hands-on Pythonic example. The goal is to institute the importance of data, especially in its worthy format, and the spell it casts on fabricating smart learning algorithms.
1:10pm ET
Designing & Operationalizing Responsible ML Systems
Ameya Diwan – Ethical AI Analyst at Rocket Central
In today’s fast-paced technological advancements, keeping ethical considerations in mind while designing and deploying artificial intelligence & machine learning systems has become increasingly crucial. In this talk, we will emphasize on the importance and need for Ethical AI. We will also identify the challenges faced by data science and machine learning professionals in designing responsible systems, and discuss solutions & best practices in depth to operationalize enterprise-wide adoption of Responsible AI.
1:40pm ET
Automated, Scalable and Quality Machine Learning with Airflow, Kubernetes, and Great Expectations
Brittney Monroe – Sr. Engineer at Carvana
Christina Taylor – Senior Staff Data Engineer at Catalyst Software
Previously, the NLP model training was a manual process. These steps included piecemeal jobs spread across multiple GCP projects with various timing/scheduling. Airflow enables us to automate the entire process on a schedule or on-demand with little to no human intervention. We can now break down a monolithic job into several dependent components. This prevents full job failure, allows us to reprocess independently, and train models faster in parallel. We further implemented GKEStartPodOperator to isolate dependencies and spin up customizable resources as needed, as well as incorporated Great Expectations for data quality checks.
2:10pm ET
15 min break
2:25pm ET
Data to Decisions, Leading with AI: A Panel Discussion
Jigyasa Grover – Senior Machine Learning Engineer at Twitter
Premalatha Thangamani – Director of Data Science at PayPal
Sharmila Chockalingam – Head of Product Marketing at Comet ML
This panel session will focus on some key topics in Machine Learning that are relevant today. These include how do you successfully take your machine learning models from research to deployment in the real world, what are the best practices there. How do you ensure good communication and collaboration across different stakeholders and business leaders to provide visibility and understanding. How to scale your machine learning efforts (both teams and projects). We will also touch upon all the latest craze around Generative AI and LLMs and see how they can be used in real world situations. What are the applications apart from creative and fun uses of these technologies.
3:10pm ET
QA for AI Systems
Serg Masís – Climate & Agronomic Data Scientist at Syngenta
Trust is mission-critical for any technology, so if AI solutions are to supplant software, AI must reach the reliability standards currently expected from software. For that to happen, a new field of MLOps engineering has branched off from the DevOps. Also, Explainable AI (XAI) will be more widely adopted since it provides the toolset to interpret machine learning predictions and scrutinize metrics. To ensure increased reliability, and robustness new roles for Machine Learning Quality Assurance will appear likely within DevOps, SecOps, and MLOps teams, but also the roles of data scientist and Machine Learning engineer will evolve.
We will examine examples and discuss how they can revolutionize the way we train, but most importantly, evaluate and deploy machine learning models with examples from the agribusiness industry and the digital agronomy field.
3:40pm ET
Optimizing Recommendations for Competing Business Objectives
Ali Vanderveld – Senior Staff Scientist at Wayfair
Recommender systems are now ubiquitous in e-commerce. At Wayfair, we use a collection of machine learning models to predict which content and which products to show our customers at every stage in their shopping journey. Models for recommendations are typically trained to optimize for near-term measures of customer satisfaction, such as conversion. However, there are other business objectives that can appear to be in conflict with this idealized ranking. For example, some products might have higher margins, and surfacing such products higher could boost long-term profit. However, such boosts will likely come with a decrease in short term conversion. Thus we need to find ways to balance these competing objectives if we want to make our product recommendations “profit aware.” In this talk I will give an overview of this problem and how we have been addressing it at Wayfair.
4:10pm ET
15 min break
4:25pm ET
Inside Stable Diffusion on CPU: Software and Hardware for AI Everywhere
Wei Li – VP and GM, Artificial Intelligence and Analytics (AIA) at Intel Corporation
We have a great opportunity to democratize AI. The challenge is that AI is very compute intensive, which often requires additional specialized AI accelerators. However, CPU is ubiquitous. With the recent built-in acceleration for AI in mainstream CPU, we have a breakthrough in making AI widely available. In this talk, we will show an amazing application, Stable Diffusion, on Intel’s latest CPU, and take a peek inside the software and hardware that make this possible.
4:55pm ET
Adopting a Data-First Approach in ML Teams for Increased Impact
Iswarya Murali – Principal Data Scientist at Microsoft
Madonna M Dodd – Principal Data Engineer at Microsoft
“Data is food for AI” – Andew Ng. According to Forbes, Data Scientists spend approximately 80% of their time collecting, cleaning and organizing data before even starting the exploratory analysis phase. This particular task could be mundane and often frustrating but is critical. Producing data that is consistent, reliable, available, and of high quality is crucial to the success of Machine Learning projects. The consequences of not investing enough into data and analytics engineering could result in feeding incomplete (or worse, incorrect) training data into models. In this talk, we’ll discuss the process of building a “data muscle” and how to incorporate engineering principles such as Scalability, User Privacy, Maintainability, Code Modularity, and Redundancy in order to create a well-functioning and impactful data team.
5:25pm ET
Wrap up
Day 2 | Wednesday March 8
12:00pm ET
Introduction
12:05pm ET
AI Operationalization - From ouch to WOW
Sandhya Gopalan – AI & MLOps Practice Head at EY Global Delivery Services
In the past few years with the rapid growth of data and increase in compute capacity, more and more companies are adopting Data Science, and ML to solve complex real-world business problems. Organizations continue to experiment and investing heavily on building capability in Data Science, Machine Learning and Artificial Intelligence. The evolution of data, computation and analytics has resulted in real time usage of data, increasing number of algorithms for experimentation, rising number of ML models for usage and management, and increasing trend of automation for each of the analytical processes.
However, industry focus has been more towards design phase of predictive analytics which is getting the models right from build, test, and validation perspective. The recent surveys have revealed that less than 40% of companies have operationalized ML models. This has hampered industry from achieving full potential of analytics. To overcome this, the next era of AI&ML is going to be more about transitioning from design phase to implementation phase covering model management, deployment and monitoring for seamless integration and smoother functioning of analytics embedded into business processes.
This talk tries to address those gaps by providing a framework and well-defined process to address these challenges help companies realize true business value.
12:40pm ET
Measuring MLOps ROI & Team Productivity
Mikiko Bazeley – Head of MLOps at Featureform
No other sentence like “”Show me the money”” sparks more dread in MLOps teams & initiatives. And yet, the premise for most MLOps tools and teams is increased productivity which should lead to a healthier bottom-line.
In this talk I’ll try to answer the following questions:
✅ What are the North Star metrics of an effective ML and MLOps practice?
✅ How do we connect the tactical, operational, & strategic layers of an MLOps practice?
✅ How should MLOps and Data Science teams be organized to provide the most value to the organization?
We’ll develop a potential framework using a combination of case studies of existing MLOps teams, evergreen wisdom from DevOps, & my own experiences traversing the ML lifecycle through a variety of industries & companies.
1:10pm ET
Data Storytelling in Comet
Angelica Lo Duca – Researcher at IIT-CNR
Data storytelling is the art of using data to tell a story, communicate insights, and invite an audience to action. Comet is an experimentation platform that enables you to track and monitor your machine learning experiments during almost the project life cycle. In Comet, data storytelling can be accomplished through panels and reports. Panels are customizable visualizations that allow users to explore and analyze data in real time. On the other hand, reports present data in a structured format, often including charts, graphs, and tables. This talk describes how to combine reports and panels in Comet to communicate the story behind data to stakeholders, making it easier to identify trends, discover insights, and drive decision-making.
1:40pm ET
How to Build and Scale AI/ML Adoption in the Financial Services Industry
Greig Cowan – Head of Data Science for Data Innovation at NatWest Group
NatWest group is one of the UK’s leading banks, serving over 19 million customers. In this talk Greig Cowan will highlight many of the challenges that NatWest faced regarding the creation of AI/ML-led solutions that are needed to unlock business value from our data. He will discuss NatWest’s approach to tackling these challenges during the past few years to create a modern cloud-based development and deployment platform that allows us to innovate more quickly, create more personalised experiences for our customers and maintaining focus on the enhanced risks that come with building and operating AI/ML-driven products.
2:10pm ET
15 min break
2:25pm ET
How Well Are You Utilizing Your GPU Clusters?
Ronen Dar – CTO at Run.ai
GPUs are crucial for ML development but are scarce and expensive and require complex scheduling, allocation and monitoring tools. In this talk we’ll discuss how AI/ML teams can better utilize their AI compute arrays and run more ML jobs without exceeding budgets.
2:55pm ET
Building Cross-functional Data Science Teams
Ozlem Yaylaci – Principal Data Scientist at JLG Industries
An increasing number of organizations are creating data science teams to recognize the potential of their data to boost performance. Since data science is a relatively new field, however, most companies have a hard time integrating their data science teams to the larger organization, and data scientists can find themselves in a lonely corner trying to manage unrealistically high expectations, without proper guidance from the business.
In this talk, we will explore best practices for creating a diverse and cross-functional data science team that can integrate to the business and harness their full potential, to the benefit of the organization.
3:25pm ET
Human-Friendly, Production-Ready Data Science with Metaflow
Hugo Bowne – Head of Developer Relations at Metaflow/Outerbound
Over the past five years, many new tools have emerged in the field of MLOps and the existing ones have matured. Yet, there is not a clear picture of a canonical stack for productive data science and ML organizations. Through our work with open-source Metaflow, which was started at Netflix in 2017, we have had an opportunity to talk to hundreds of companies at various levels of maturity when it comes to ML infrastructure. In this talk, we will provide an overview of key pain points that we have observed – both technical and organizational – and suggest how the evolving landscape of tooling can help address these challenges. We’ll also discuss the need for interoperable tools in the space, which motivated Metaflow’s recent integration with Comet.
3:55pm ET
Kangas: Visualizing Mixed Multimedia Data at Scale
Doug Blank – Head of Research at Comet
Caleb Kaiser – Software Engineer at Comet
In this talk we introduce a new open-source project called Kangas that allows easy exploration and analysis of data when it is mixed with large multimedia data types, such as images, video, and audio.
4:20pm ET
Wrap up
Past attendees from
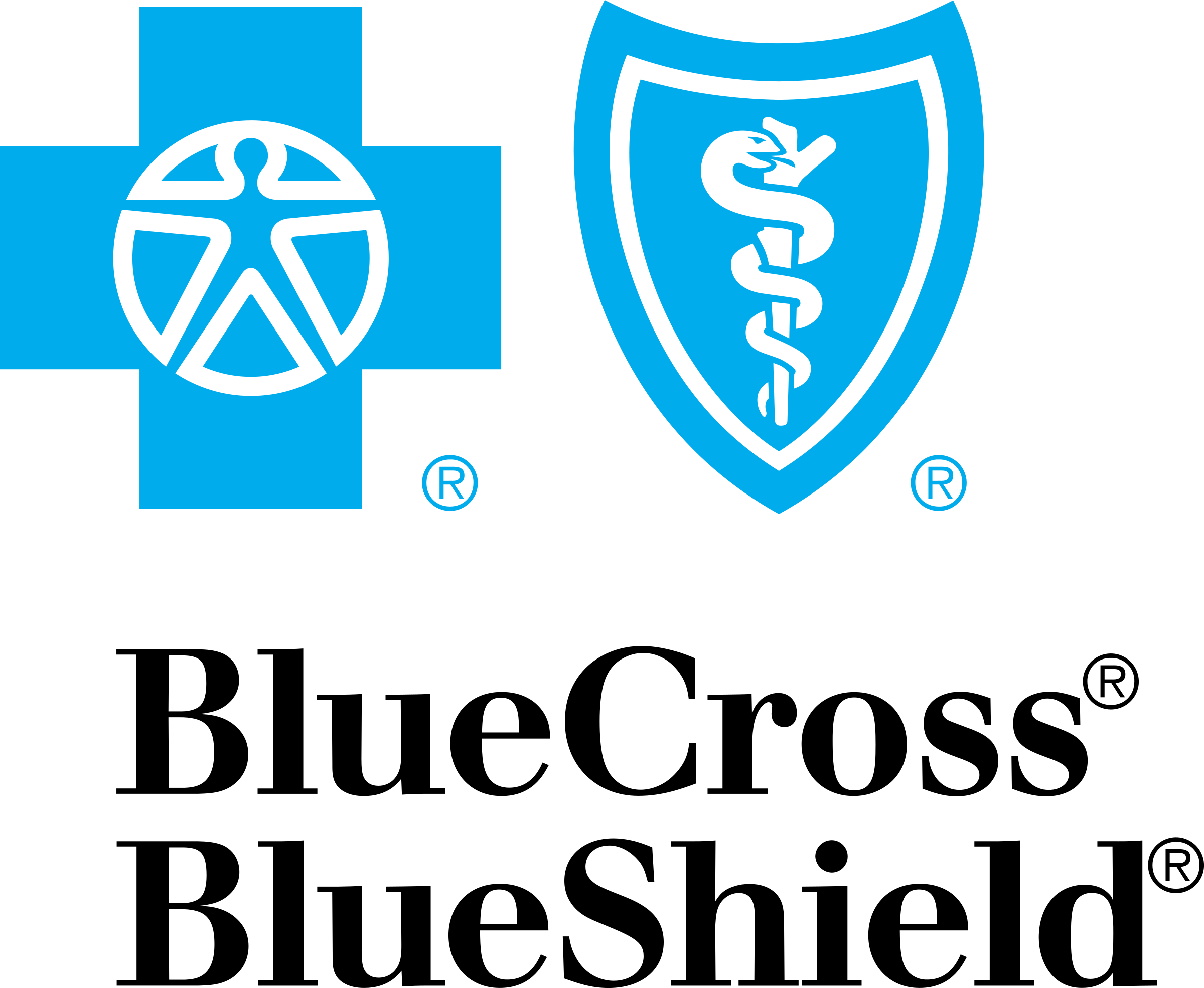
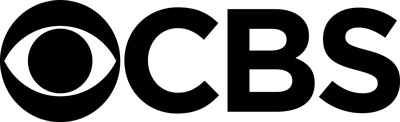
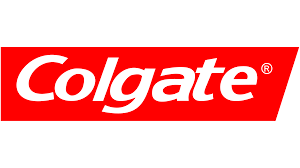
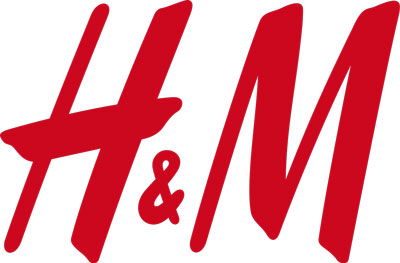
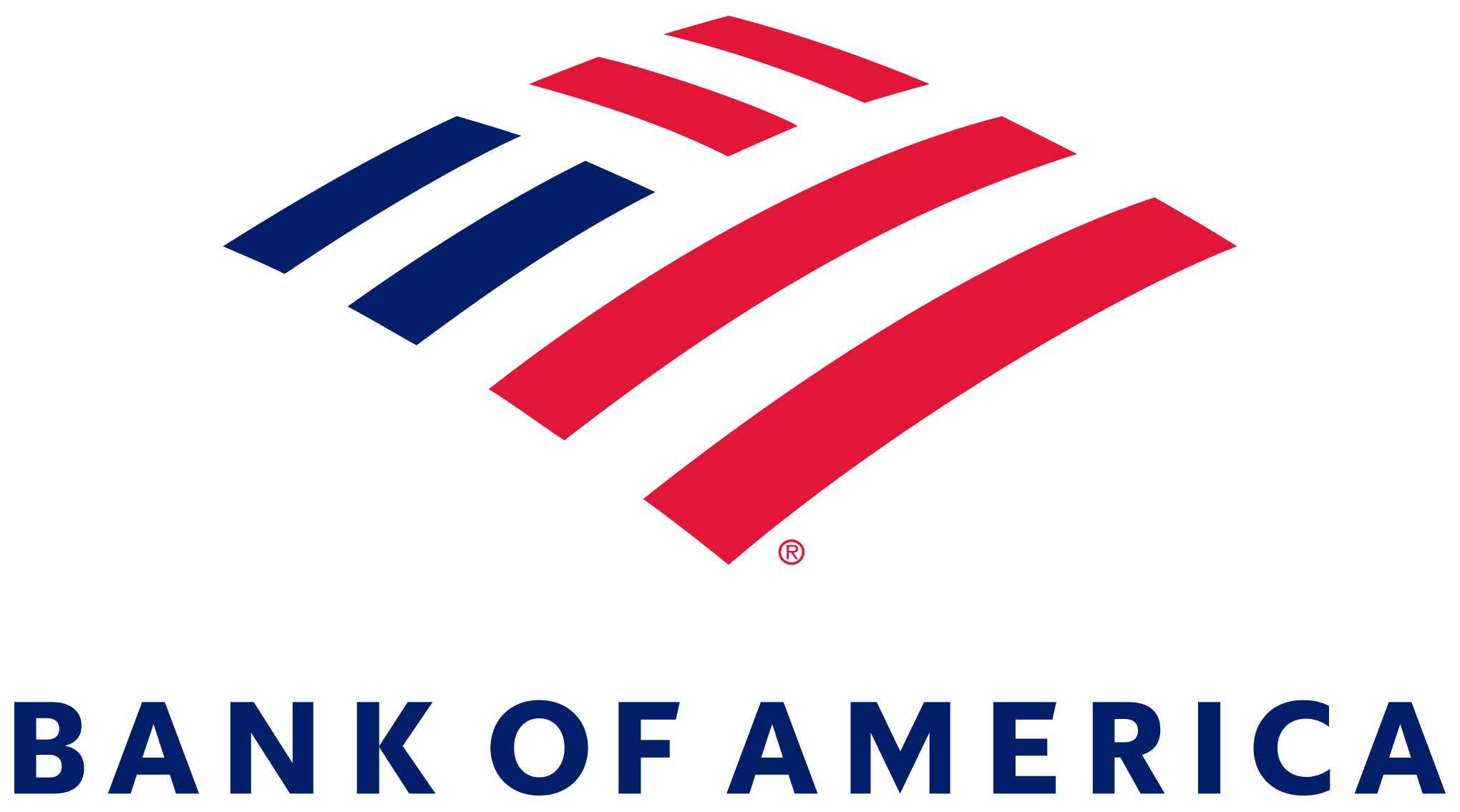
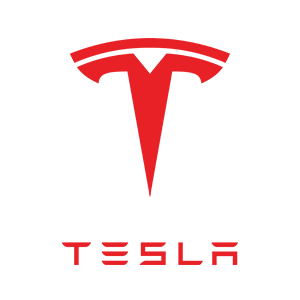

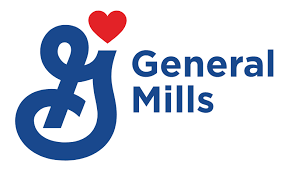
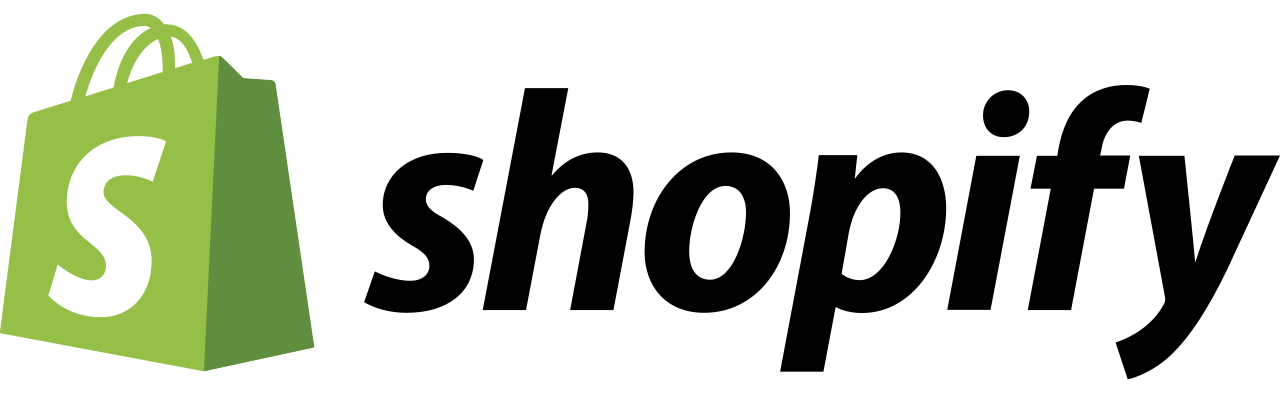
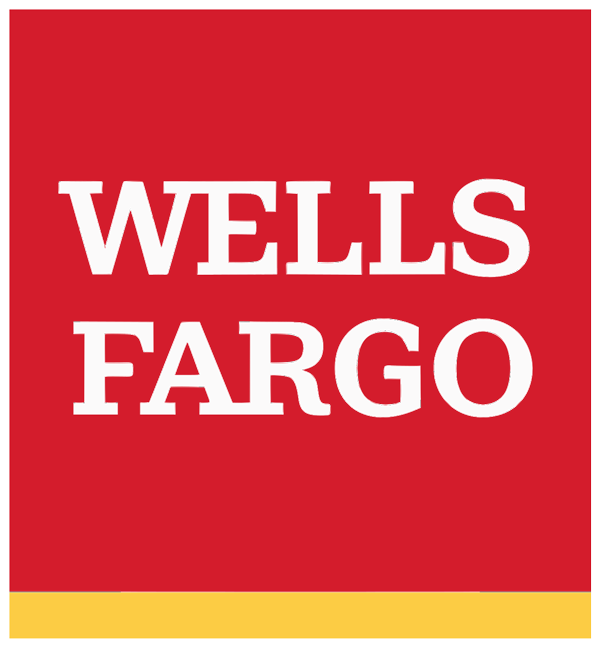

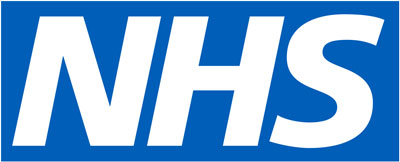

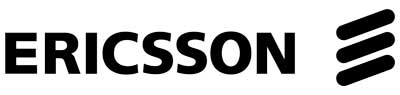

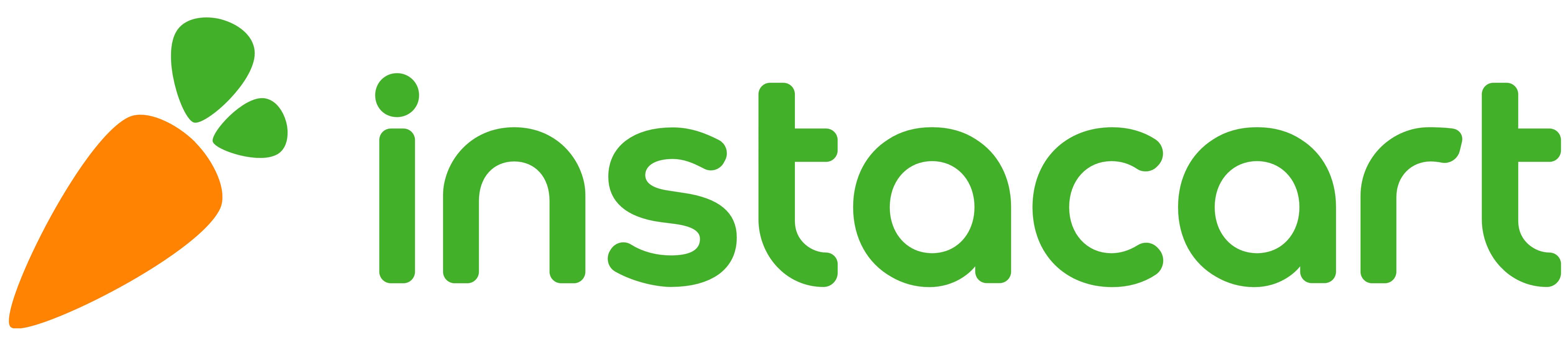
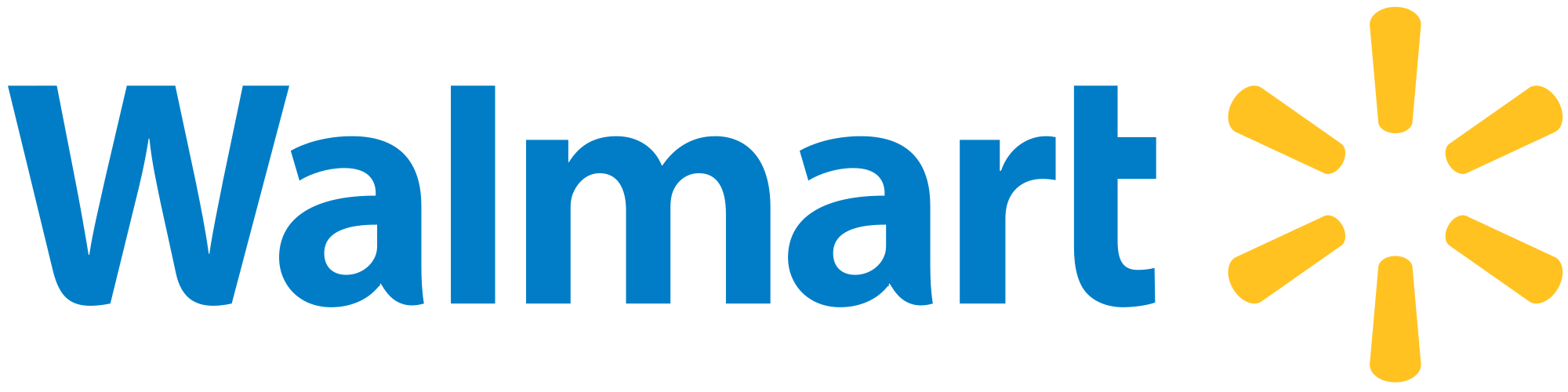